Guardian Fleet Monitoring – 3rd Generation
Drivers live in their trucks in a way few others understand. They deal with varied weather and stress, balancing time and safety pressures. Fatigue and distraction are the most critical safety risks.
Guardian is an after-market camera system that uses both AI and human review to flag distraction and drowsiness in fleet transportation drivers to prevent accidents before they happen.
Good design should support decision-making by both drivers and management based on actual performance, not arbitrary timetables. Management needs data to shape proactive strategies. Drivers need to know that responsible behavior will be protected and rewarded.
Since 2005, Guardian has tracked:
- 15 billion kilometers of real-world, naturalistic driving
- 17.6 million distraction events
- 390 thousand fatigue interventions per day
The 3rd-generation Guardian service system design updates the tracking algorithm to best in class, automotive-grade algorithms to provide more mature tracking to increase accuracy, decrease hassle alarms for drivers, and improve the scalability of human reviewer staffing.
Outcome
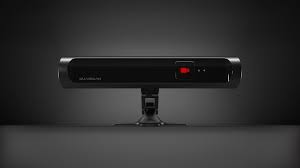
Released at CES 2024, Gen 3 provides:
- The first automotive-grade fatigue and distraction detection AI provides:
- Multiglance detection independent of any single glance
- Cumulative fatigue detection rather than an eye-closure timer
- A gradual, multi-sensory interface that builds in severity, rather than the “shock-and-awe” approach of immediate, strong vibrations and loud alerts, startling frustrated drivers.
- Full eye-tracking that doesn’t penalize drivers for mirror-checking
- Tracks real people in real environments: low light, around masks, heavy glasses, beards, make-up, road glare, shadows, etc.
- World-wide, human-in-the-loop, 24/7 SaaS monitoring and intervention
- 30-minute after-market installation
- New fleet trucks are available with pre-installed hardware, fitted immediately after the assembly line
- Combined visual, auditory, and vibrotactile icons to communicate status to drivers with minimal interruption
- Driver privacy for any non-event-related data, encouraging fleets to strategically, proactively protect driver safety
Project at a Glance
My role:
- Translate bleeding-edge distraction and fatigue algorithms into system architecture requirements
- Research and data analysis support to support design decisions
Timeframe: 3 months
Challenges Faced
- Previous systems were based on detecting single events, such as an eye closure longer than 3 seconds. Moving to cumulative events created new problems:
- How do you package up 30 minutes of fatigue behavior for quick, reliable human review?
- How do you train reviewers for the new format?
- How do you reduce the workload for reviewers to improve scalability?
- If both fatigue and distraction algorithms run simultaneously, which events are a priority?
- If connectivity fails, what events need to be saved, and which should be deleted?
- How should we structure in-cabin alerts and escalation to improve driver behavior?
- How can we use all of a driver’s senses to improve understanding of alert severity, even under distraction and stress?
- COVID-era project limitations: the team had to constantly reassess designs based on what suppliers were available or not.
Insights Discovered
- Data Analysis: Eye closure, alone, doesn’t equal drowsiness. 90% of eye-closure events longer than 2 seconds are due to non-drowsy causes (e.g.: rubbing sore eyes, singing with the radio, squinting due to glare, etc.)
- Data analysis and user testing: Specified how much time video reviewers needed to make a ruling on whether or not to intervene in cumulative drowsiness or multi-glance distraction events
Skills Used:
- Data analysis
- Interface design: unified visual, auditory, and vibrotactile icons
- User performance studies
- Evaluation of algorithm performance
- System design
- Video editing